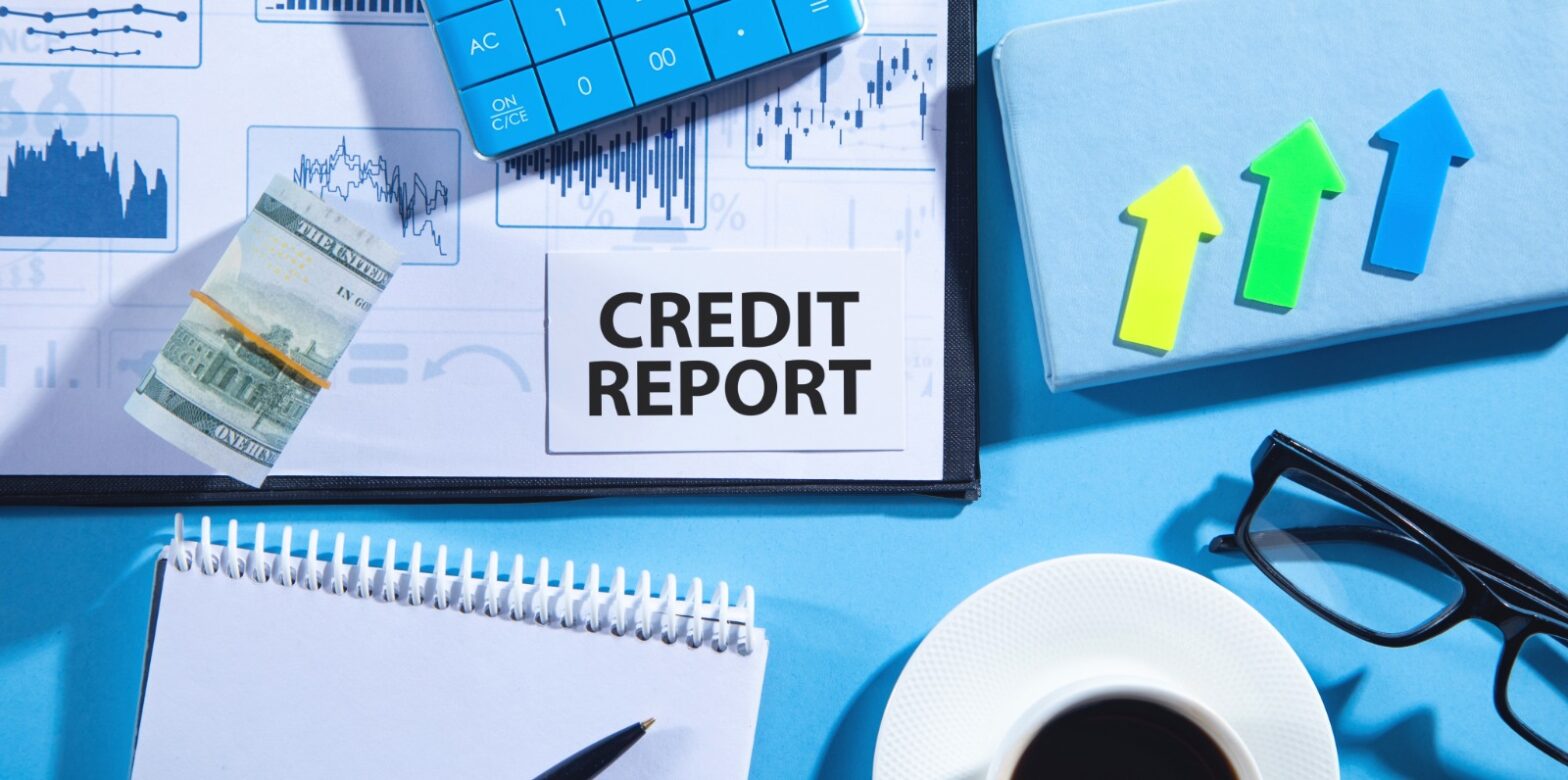
Assessing the credit default risk of the borrower and keeping an eye on their behavior has been a key responsibility of the risk monitoring department in numerous banks and financial institutions. Credit frauds totaling thousands of crores and major economic crises (such as global crisis in 2008) show the impact of inadequate risk monitoring. Considering such events, RBI carried out circular in year 2016 as an extension of fraud monitoring listing indicative scenarios to throw early warnings of credit fraud or potential defaults. With this circular, Credit Monitoring for high-risk exposure accounts became a regulatory requirement for banks, non-compliance of which would result in penalties.
However, Credit default and fraud predictions become more challenging without an automated intelligent credit monitoring system in place, that can harness the power of digitization.
Some of the impacts due to absence of an effective solution can be:
- Delays in Credit Risk Assessments and risk mitigation
- More susceptibility to Credit Frauds
- Financial and Reputational Losses
- Penalties for Non-Compliance
- Lack of profound insights and inadequate interpretation of borrower’s activities
- Increased personnel expenditures for risk management
- Incapacitated data collection essential for monitoring.
The above shortcomings without an all-encompassing solution establish the critical need for an organization to have a digital platform that expedites and improves early detection of potential credit frauds, cases like willful defaults, and losses due to NPA.
Challenges and Expectations from Credit Monitoring Solutions
Although it’s normal to expect great things out of technology and digitalization in terms of ROI, data is foundation of any analytics-based platform, which is one of the key challenges for an EWS solution. “Garbage in garbage out” is a common term denoting this dependency. Thanks to advancements in data exploration, processing, analysis, and analytics in recent years, let’s see some of the key requirements an advanced EWS solution must fulfil-
Tackling Diversities of portfolio and variety of facilities
Solution is expected to handle underlying data requirements to monitor all types of borrowers such as Retail, MSME, Large Corporate borrowers. It should expand integration capabilities to provide customer 360 and comprehensive risk indicators to monitor different facilities such as retail loans, working capitals, Bank Guarantees, Letter of Credit, Factoring and Re-financing Services. Pre-configured integration with some of the promising information sources should be:
- Banking source channels such as CBS, Trade Finance, LOS
- Multiple external APIs such as MCA, GST, CIBIL, Audit and Research report, Criminal Background Checks
- Insights on negative sentiments for a borrower or an industry from web media.
- Impact of macro-economic factors and sectoral dependencies
- Un-conventional Information Sources
Many of the information sources for EWS are external sources and several of them are un-structured such as web media, financial reports, financial and stock statements. Therefore, latest techniques like text mining, web scraping and API integrations are key features for a comprehensive EWS solution to offer.
Identifying critical use-cases like willful defaults and siphoning of funds –
One of the primary expectations from EWS is to leverage advanced analytical techniques for detection of critical fraud use-cases such as siphoning of funds transaction with shell companies and related party transactions. These are some methods which can be carried out either to fake financial health conditions, or mis-use credit facilities. Most corporate scams include some of these scenarios as main method.
A unique feature to extract insights about related parties and their funding is graph based linkage analysis. Use graph to explore company directors, other directorships, transaction summary between buyers/suppliers and un-related parties. Multiple parties exchanging funds in multi layers or exhibiting other important scenarios such as common guarantors or collateral can be easily visualized using graph technology.
Artificial Intelligence
An advanced EWS solution is infused with data science and business analytics to improvise delinquency predictions and optimize processes backed up with statistical analysis. Some inherent features leveraging statistical techniques would be
- Machine learning models to complement business rules for AI based delinquency predictions.
- Decision points in product such as threshold tuning, case investigation are backed up with statistical analysis.
- Management dashboards to highlight stress on portfolio across dimensions of industry and facilities.